Table of Contents
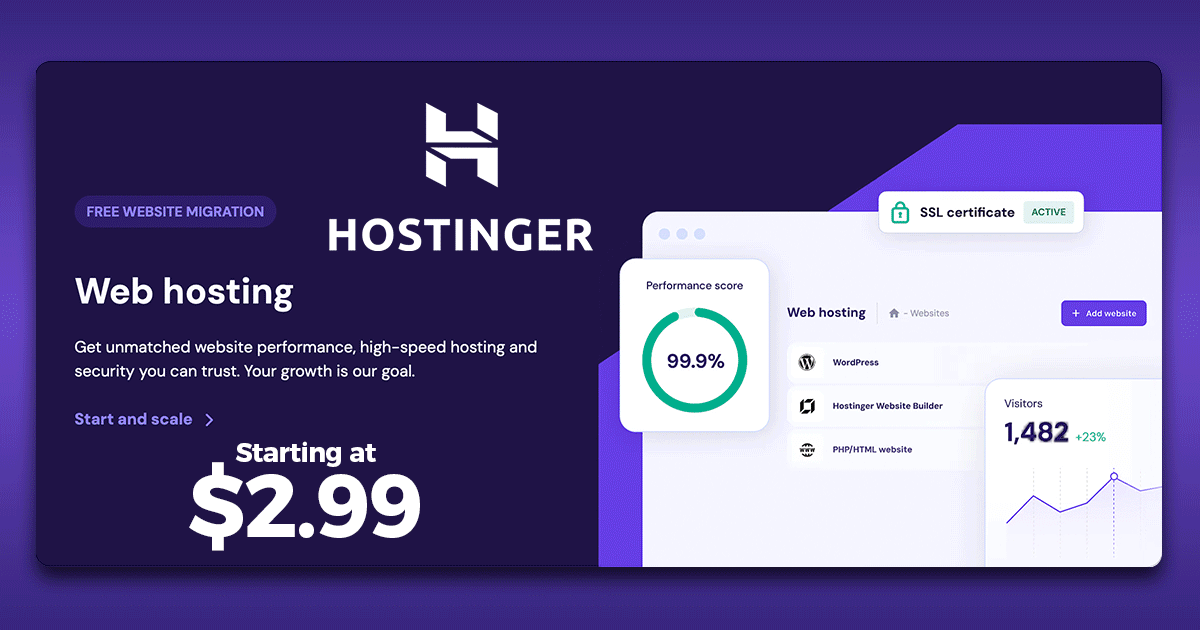
What is happening with Digital Twins in The Metaverse?
The industrial metaverse is emerging as a transformative force that blends the digital and physical realms, reshaping sectors such as manufacturing, healthcare, and energy. With the ability to conduct real-time process simulations and predictive maintenance, it empowers businesses to enhance efficiency, lower costs, and foster unparalleled innovation. Central to this shift are Digital Twins (DTs)—virtual models of physical assets that replicate their behavior in the real world. However, as industries adopt DTs within their workflows, a pressing question arises: How do we ensure the security and authenticity of these digital replicas?
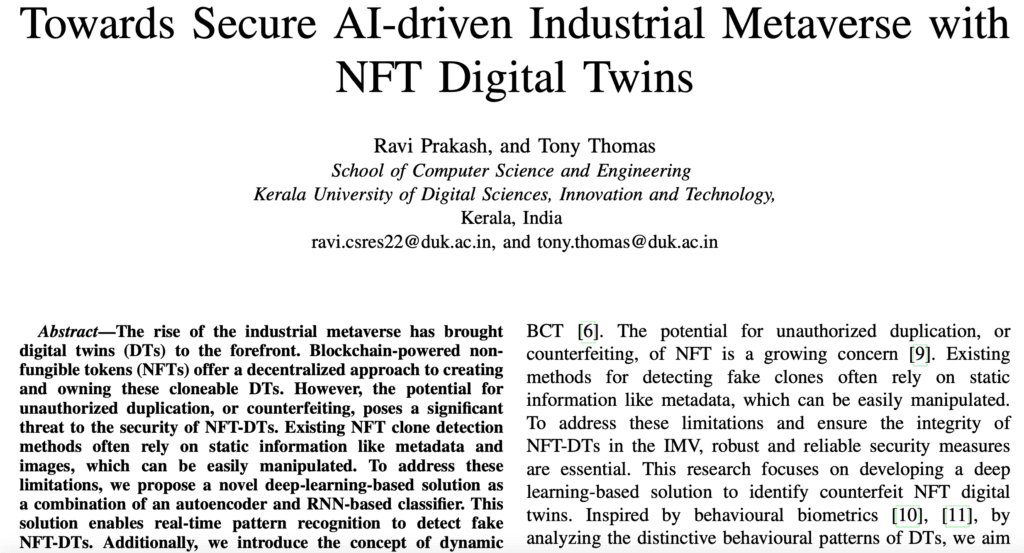
A research paper titled “Towards Secure AI-driven Industrial Metaverse with NFT Digital Twins,” currently accessible on arXiv, explores the increasing challenges of protecting Digital Twins, particularly those verified through Non-Fungible Tokens (NFTs). The existence of counterfeit DTs not only jeopardizes operational productivity but also creates significant risks of industrial espionage and process breakdowns.
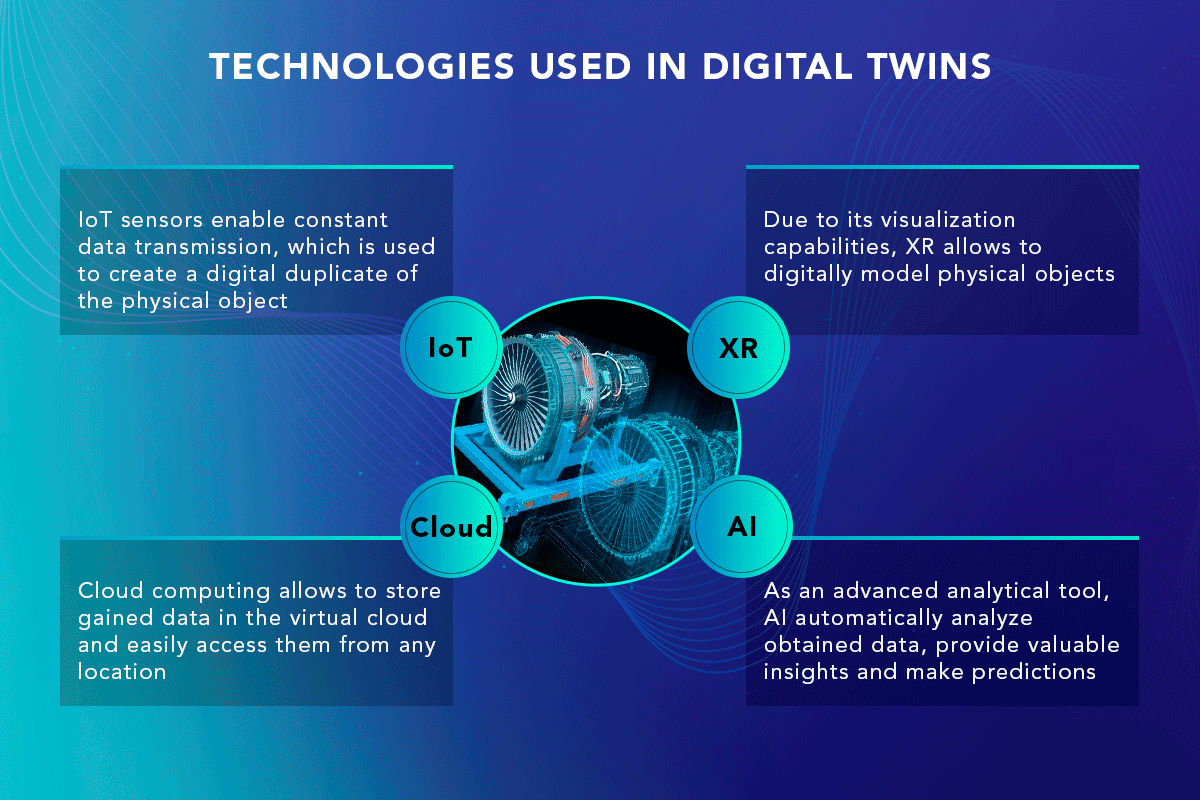
This study introduces an innovative approach to safeguard NFT-based DTs against counterfeiting, a vulnerability that threatens the integrity of the industrial metaverse. By harnessing advanced AI techniques and dynamic metadata, the proposed system establishes a benchmark for securing the future of digital twins.
The industrial metaverse: Opportunity meets risk
The industrial metaverse presents tremendous potential. By 2030, the global digital twin market is projected to reach $73.5 billion, driven by sectors like manufacturing, energy, and healthcare leading the way. DTs enable businesses to streamline operations, explore new strategies, and anticipate equipment failures—all without physical intervention. For instance, a wind farm operator can utilize DTs to oversee turbine performance, pinpointing potential problems before they escalate into failures. Likewise, car manufacturers can run crash test simulations with DTs, expediting the design process and diminishing the costs of physical prototypes.

Nonetheless, the inclusion of DTs in critical business functions carries inherent risks. NFTs, often seen as a means to authenticate ownership and identity of digital assets, have gained popularity for managing DTs. While NFTs provide traceability and distinctiveness, they are not immune to counterfeiting. Malicious actors can replicate or alter NFT-based DTs, introducing vulnerabilities that threaten the entire metaverse ecosystem.
The researchers in the study underscore how such counterfeit copies could disrupt industrial functions. For example, a fake DT of a factory’s robotic arm might transmit inaccurate data, causing costly inefficiencies or, even worse, safety risks.
Fighting counterfeiting with AI and dynamic metadata
The study advocates for a pioneering solution to combat counterfeit DTs through AI-driven dynamic metadata and behavioral pattern analysis. Unlike the static metadata typically employed in NFTs, dynamic metadata adapts in real time, monitoring alterations in the DT’s operating parameters. This ensures immediate detection of any tampering attempts.
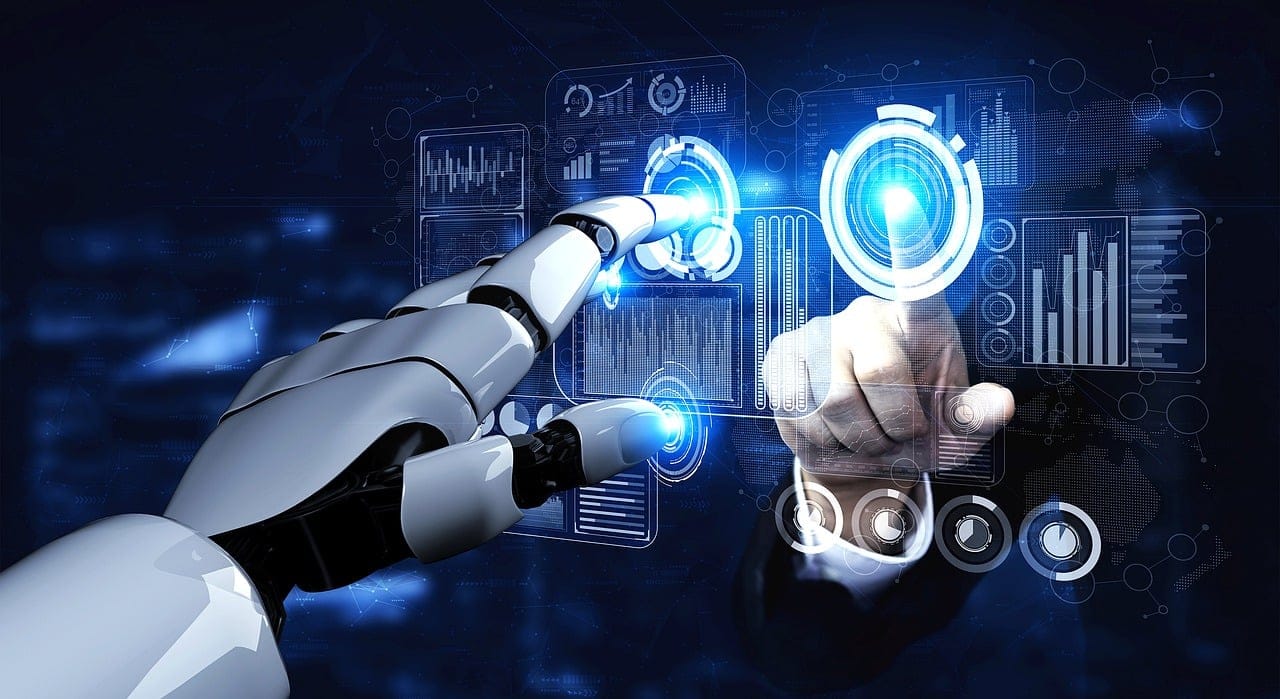
Central to this innovation are Denoising Autoencoders (DAE) and Bidirectional Gated Recurrent Units (Bi-GRU). These deep learning models scrutinize the behavioral characteristics of DTs, accurately differentiating between genuine entities and fraudulent clones.
During their experiments, the researchers evaluated the proposed framework using a simulated dataset involving heaters, achieving remarkable results. The system displayed an impressive accuracy rate of 98.3%, reliably distinguishing authentic digital twins from counterfeit ones with extraordinary precision. The incorporation of dynamic metadata and AI technologies fortified the framework against tampering, rendering it nearly impossible for attackers to circumvent detection systems.

Moreover, the framework bolstered operational security by implementing real-time updates and behavioral oversight, which not only reduced false positives but also ensured effective management of potential risks. These findings highlight the framework’s potential to emerge as a dependable solution for securing digital twins in the industrial metaverse.
Securing the future of digital twins
As the industrial metaverse expands, ensuring the authenticity and security of Digital Twins becomes imperative. The impact of the proposed framework reaches far beyond manufacturing. In healthcare, counterfeit DTs of medical devices could result in diagnostic mistakes, while in urban planning, fake DTs of infrastructure assets may lead to subpar city designs. Securing DTs with AI-driven methods could help avert such outcomes, making the metaverse a safer and more trustworthy environment for innovation.
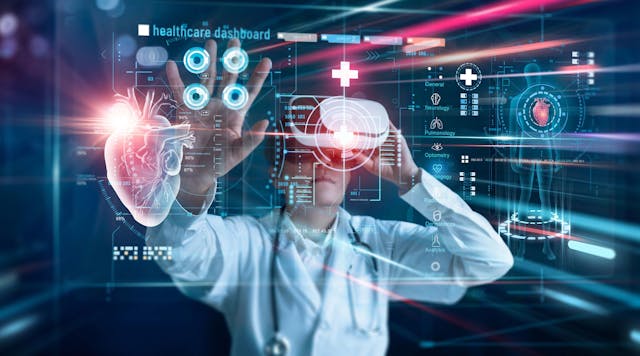
The industrial metaverse, with its promise of efficiency and innovation, stands at a pivotal moment. While the opportunities are vast, the associated risks cannot be overlooked. The study emphasizes that proactive security measures, such as dynamic metadata and AI-enhanced behavioral analytics, can lay the groundwork for a reliable metaverse. With global investment in metaverse technologies escalating, addressing these issues is both necessary and urgent.
By embracing advanced security frameworks, industries can ensure the metaverse fulfills its promise: a seamlessly integrated world where the physical and digital coexist, propelling progress and growth without sacrificing trust.